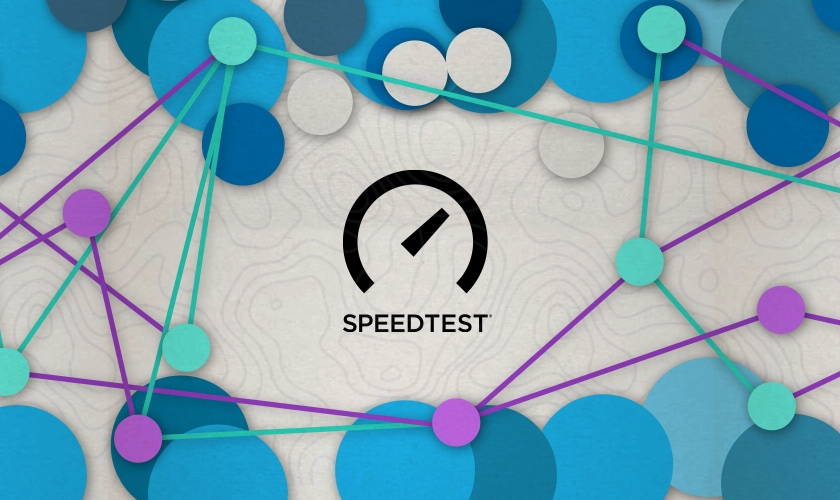
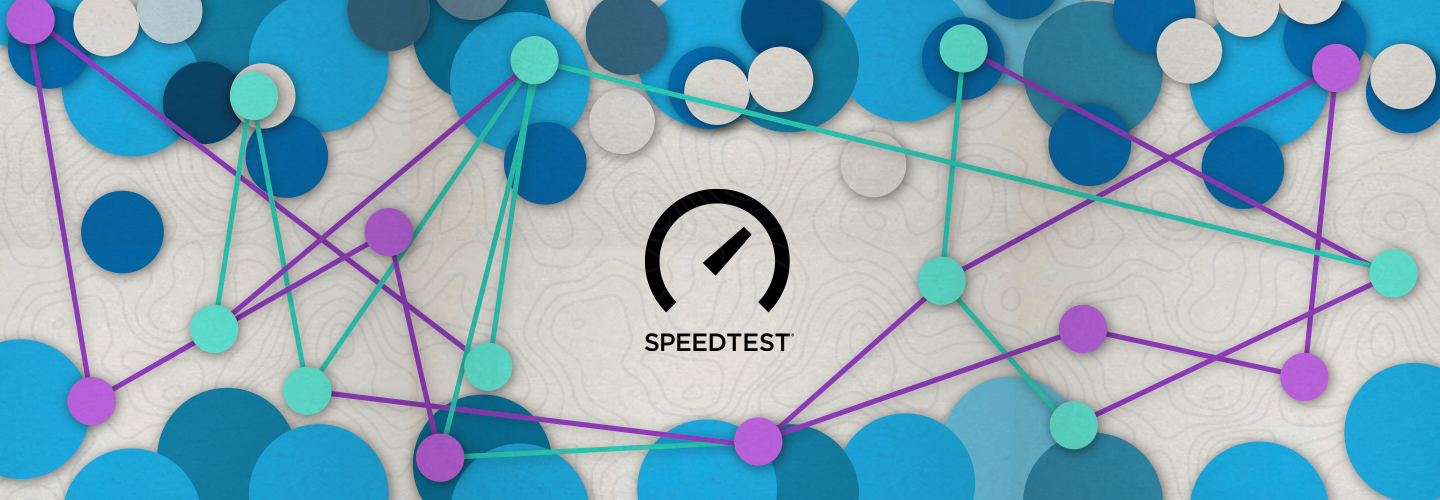
When we announced Ookla® Open Datasets from Ookla For Good™ in October, we were hoping to see exciting projects that raise the bar on the conversation about internet speeds and accessibility — and you delivered. From analyses of internet inequity in the United States to measures of data affluence in India, today we’re highlighting four projects that really show what this data can do. We also have a new, simpler tutorial on how you can use this data for your own efforts to improve the state of networks worldwide.
Highlighting the digital divide in the U.S.
Jamie Saxon with the Center for Data and Computing at the University of Chicago married Ookla data on broadband performance with data from the American Community Survey to create interactive maps of the digital divide in 20 U.S. cities. These maps provide views into many variables that contribute to internet inequities.
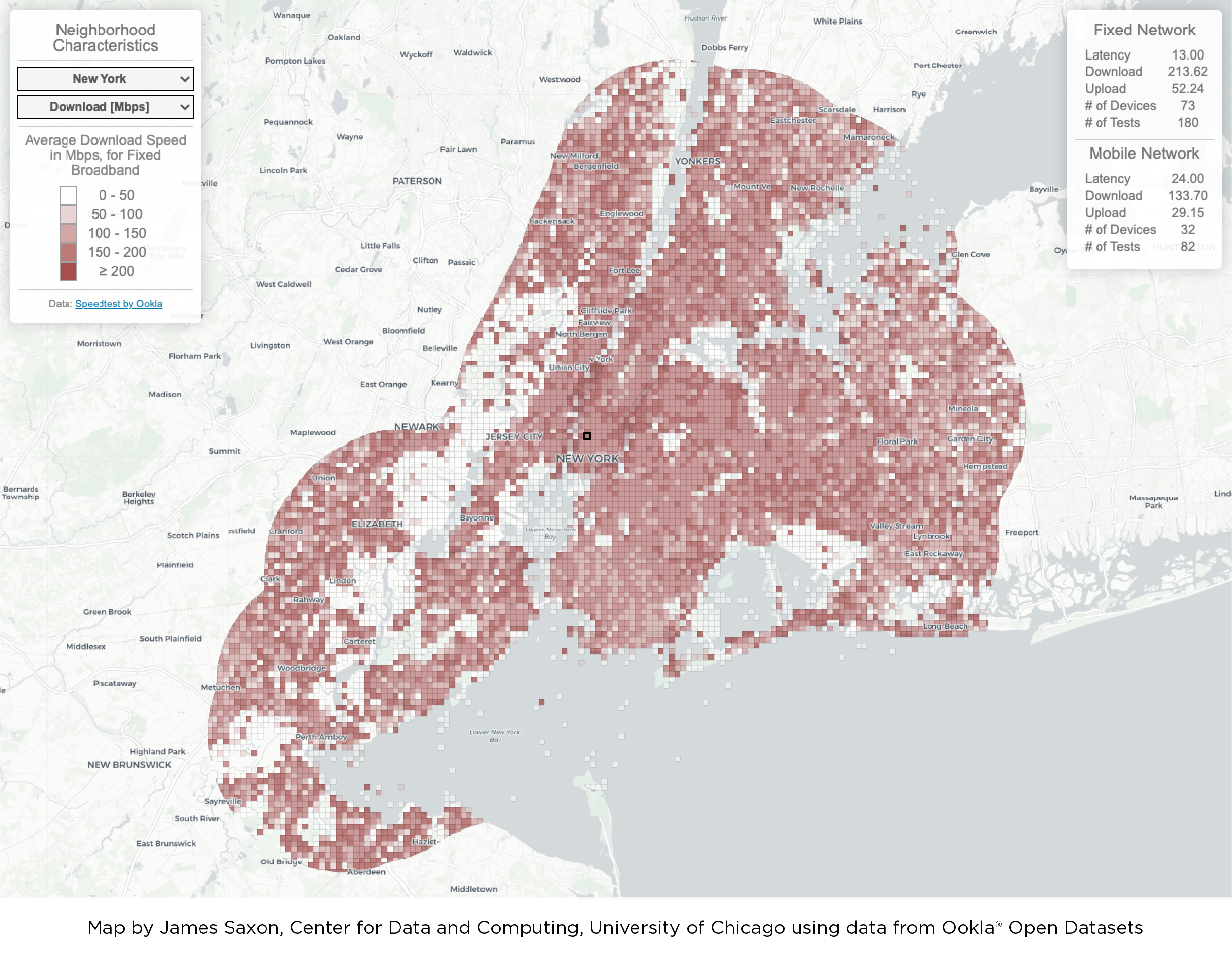
Building a data affluence map
Raj Bhagat P shows how different variables can be combined with this map of data affluence that combines data on internet speeds and device counts in India.
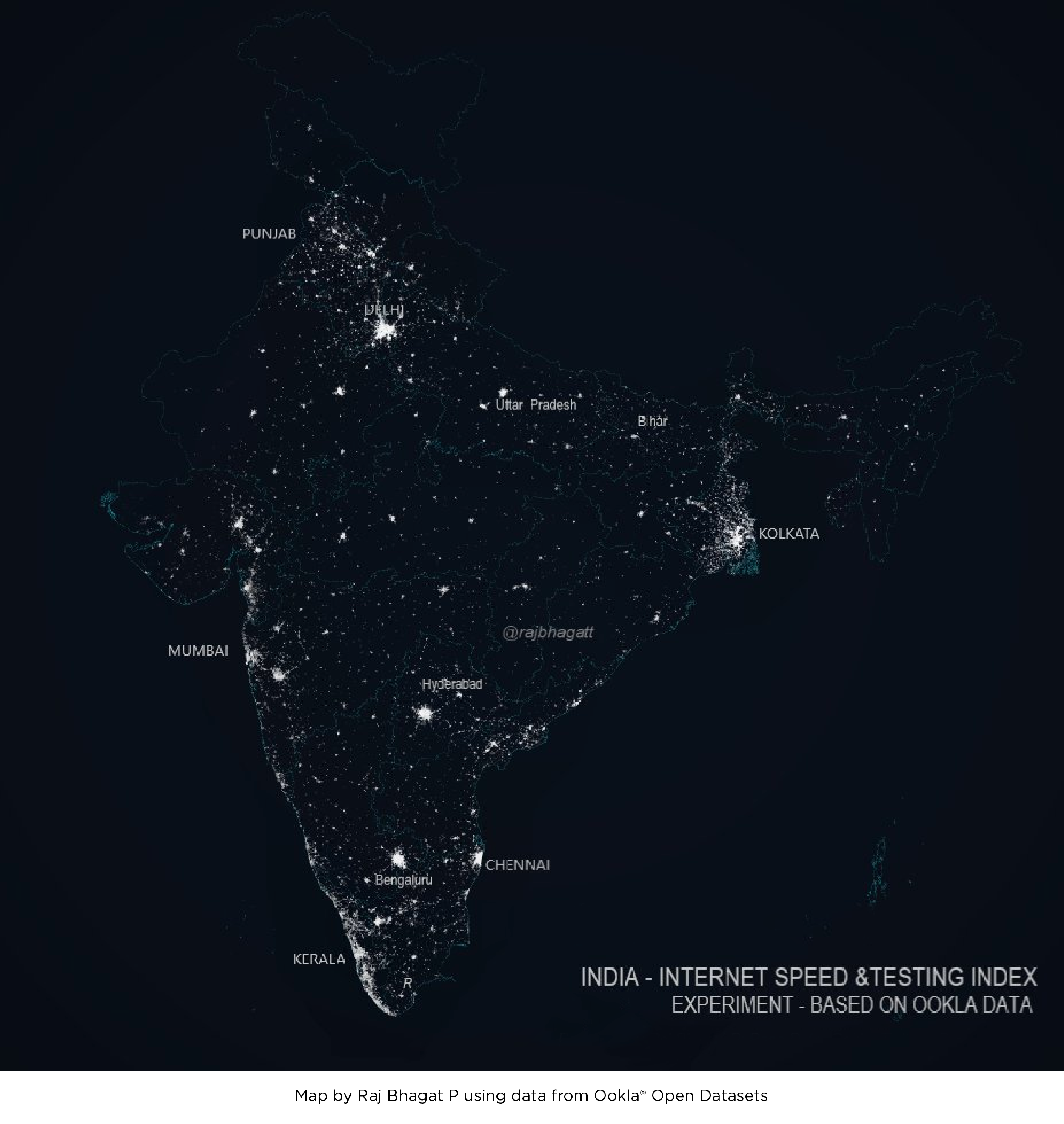
Internet speeds are beautiful
This map of fixed broadband speeds across Europe from Boris Mericskay shows that internet performance can be as visually stunning as a map of city lights.
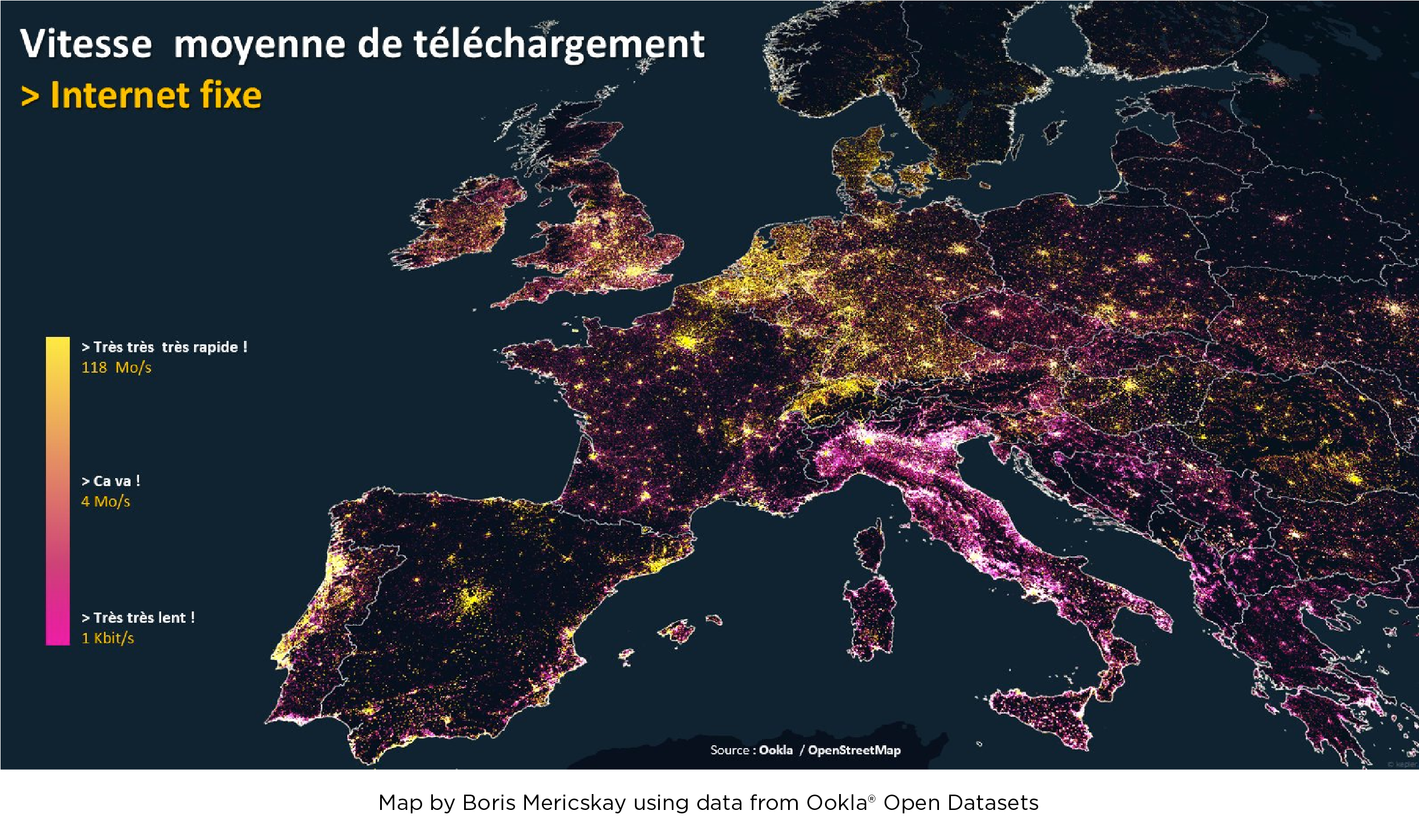
Topi Tjunakov created a similar image of internet speeds in and around Japan.
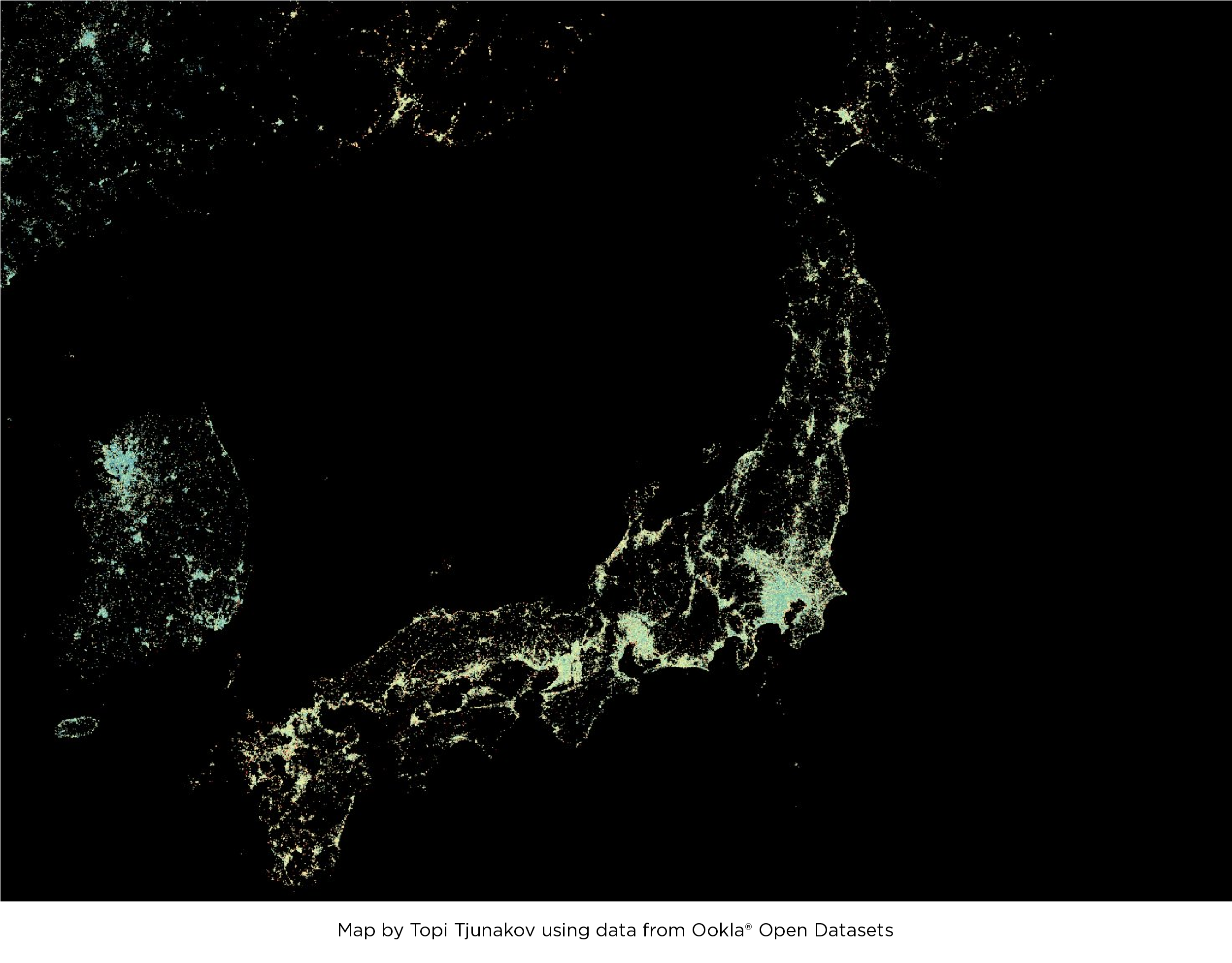
Use Ookla Open Datasets to make your own maps
This section will demonstrate a few possible ways to use Ookla Open Datasets using the United Kingdom as an example. The ideas can be adapted for any area around the world. This tutorial uses the R programming language, but there are also Python tutorials available in the Ookla Open Data GitHub repository.
library(tidyverse)
library(patchwork)
library(janitor)
library(ggrepel)
library(usethis)
library(lubridate)
library(colorspace)
library(scales)
library(kableExtra)
library(knitr)
library(sf)
# colors for plots
purple <- "#A244DA"
light_purple <- colorspace::lighten("#A244DA", 0.5)
green <- colorspace::desaturate("#2DE5D1", 0.2)
blue_gray <- "#464a62"
mid_gray <- "#ccd0dd"
light_gray <- "#f9f9fd"
# set some global theme defaults
theme_set(theme_minimal())
theme_update(text = element_text(family = "sans", color = "#464a62"))
theme_update(plot.title = element_text(hjust = 0.5, face = "bold"))
theme_update(plot.subtitle = element_text(hjust = 0.5))
Ookla Open Datasets include quarterly performance and test count data for both mobile networks and fixed broadband aggregated over all providers. The tests are binned into global zoom level 16 tiles which can be thought of as roughly a few football fields. As of today, all four quarters of 2020 are available and subsequent quarters will be added as they complete.
Administrative unit data
I chose to analyse the mobile data at the Nomenclature of Territorial Units for Statistics (NUTS) 3 level (1:1 million). These administrative units are maintained by the European Union to allow for comparable analysis across member states. NUTS 3 areas mean:
- In England, upper tier authorities and groups of unitary authorities and districts
- In Wales, groups of Principal Areas
- In Scotland, groups of Council Areas or Islands Areas
- In Northern Ireland, groups of districts
To make a comparison to the U.S. administrative structure, these can be roughly thought of as the size of counties. Here is the code you’ll want to use to download the NUTS shapefiles from the Eurostat site. Once the zipfile is downloaded you will need to unzip it again in order to read it into your R environment:
# create a directory called “data”
dir.create("data")
use_zip("https://gisco-services.ec.europa.eu/distribution/v2/nuts/download/ref-nuts-2021-01m.shp.zip", destdir = "data")
uk_nuts_3 <- read_sf("data/ref-nuts-2021-01m.shp/NUTS_RG_01M_2021_3857_LEVL_3.shp/NUTS_RG_01M_2021_3857_LEVL_3.shp") %>%
filter(CNTR_CODE == "UK") %>%
st_transform(4326) %>%
clean_names() %>%
mutate(urbn_desc = case_when( # add more descriptive labels for urban variable
urbn_type == 1 ~ "Urban",
urbn_type == 2 ~ "Intermediate",
urbn_type == 3 ~ "Rural"
),
urbn_desc = factor(urbn_desc, levels = c("Urban", "Intermediate", "Rural")))
# contextual city data
uk_cities <- read_sf("https://opendata.arcgis.com/datasets/6996f03a1b364dbab4008d99380370ed_0.geojson") %>%
clean_names() %>%
filter(fips_cntry == "UK", pop_rank <= 5)
ggplot(uk_nuts_3) +
geom_sf(color = mid_gray, fill = light_gray, lwd = 0.08) +
geom_text_repel(data = uk_cities,
aes(label = city_name, geometry = geometry),
family = "sans",
color = blue_gray,
size = 2.2,
stat = "sf_coordinates",
min.segment.length = 2) +
labs(title = "United Kingdom",
subtitle = "NUTS 3 Areas") +
theme(panel.grid.major = element_blank(),
panel.grid.minor = element_blank(),
axis.text = element_blank(),
axis.title = element_blank())
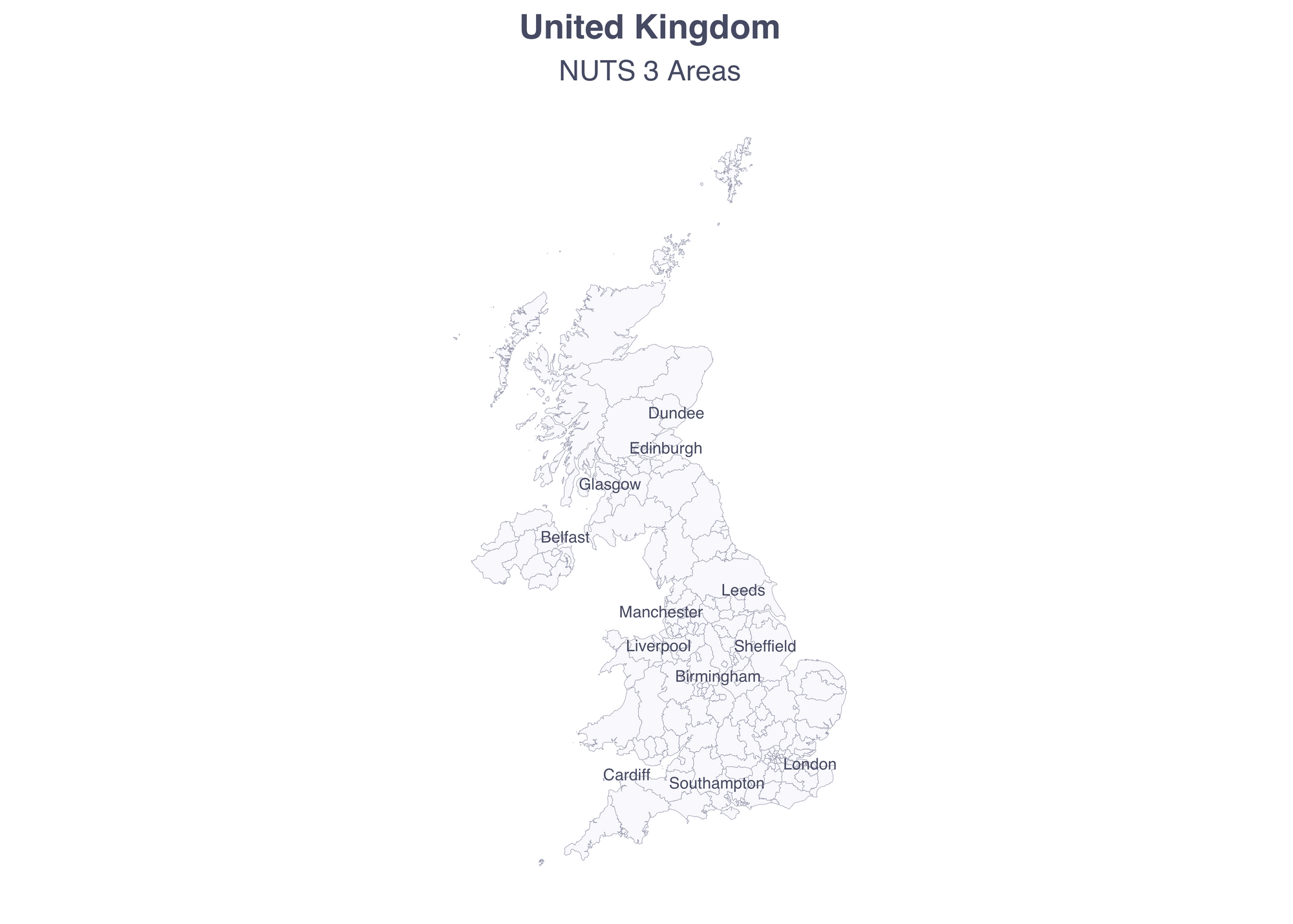
Adding data from Ookla Open Datasets
You’ll want to crop the global dataset to the bounding box of the U.K. This will include some extra tiles (within the box but not within the country, i.e. some of western Ireland), but it makes the data much easier to work with later on.
uk_bbox <- uk_nuts_3 %>%
st_union() %>% # otherwise would be calculating the bounding box of each individual area
st_bbox()
Each of the quarters are stored in separate shapefiles. You can read them in one-by-one and crop them to the U.K. box in the same pipeline.
# download the data with the following code:
use_zip("https://ookla-open-data.s3.amazonaws.com/shapefiles/performance/type=mobile/year=2020/quarter=1/2020-01-01_performance_mobile_tiles.zip", destdir = "data")
use_zip("https://ookla-open-data.s3.amazonaws.com/shapefiles/performance/type=mobile/year=2020/quarter=2/2020-04-01_performance_mobile_tiles.zip", destdir = "data")
use_zip("https://ookla-open-data.s3.amazonaws.com/shapefiles/performance/type=mobile/year=2020/quarter=3/2020-07-01_performance_mobile_tiles.zip", destdir = "data")
use_zip("https://ookla-open-data.s3.amazonaws.com/shapefiles/performance/type=mobile/year=2020/quarter=4/2020-10-01_performance_mobile_tiles.zip", destdir = "data")
# and then read in those downloaded files
mobile_tiles_q1 <- read_sf("data/2020-01-01_performance_mobile_tiles/gps_mobile_tiles.shp") %>%
st_crop(uk_bbox)
mobile_tiles_q2 <- read_sf("data/2020-04-01_performance_mobile_tiles/gps_mobile_tiles.shp") %>%
st_crop(uk_bbox)
mobile_tiles_q3 <- read_sf("data/2020-07-01_performance_mobile_tiles/gps_mobile_tiles.shp") %>%
st_crop(uk_bbox)
mobile_tiles_q4 <- read_sf("data/2020-10-01_performance_mobile_tiles/gps_mobile_tiles.shp") %>%
st_crop(uk_bbox)
As you see, the tiles cover most of the area, with more tiles in more densely populated areas. (And note that you still have tiles included that are outside the boundary of the area but within the bounding box.)
ggplot(uk_nuts_3) +
geom_sf(color = mid_gray, fill = light_gray, lwd = 0.08) +
geom_sf(data = mobile_tiles_q4, fill = purple, color = NA) +
geom_text_repel(data = uk_cities,
aes(label = city_name, geometry = geometry),
family = "sans",
color = blue_gray,
size = 2.2,
stat = "sf_coordinates",
min.segment.length = 2) +
labs(title = "United Kingdom",
subtitle = "Ookla® Open Data Mobile Tiles, NUTS 3 Areas") +
theme(panel.grid.major = element_blank(),
panel.grid.minor = element_blank(),
axis.text = element_blank(),
axis.title = element_blank())

Now that the cropped tiles are read in, you’ll use a spatial join to determine which NUTS 3 area each tile is in. In this step, I am also reprojecting the data to the British National Grid (meters). I’ve also added a variable to identify the time period (quarter).
tiles_q1_nuts <- uk_nuts_3 %>%
st_transform(27700) %>% # British National Grid
st_join(mobile_tiles_q1 %>% st_transform(27700), left = FALSE) %>%
mutate(quarter_start = "2020-01-01")
tiles_q2_nuts <- uk_nuts_3 %>%
st_transform(27700) %>%
st_join(mobile_tiles_q2 %>% st_transform(27700), left = FALSE) %>%
mutate(quarter_start = "2020-04-01")
tiles_q3_nuts <- uk_nuts_3 %>%
st_transform(27700) %>%
st_join(mobile_tiles_q3 %>% st_transform(27700), left = FALSE) %>%
mutate(quarter_start = "2020-07-01")
tiles_q4_nuts <- uk_nuts_3 %>%
st_transform(27700) %>%
st_join(mobile_tiles_q4 %>% st_transform(27700), left = FALSE) %>%
mutate(quarter_start = "2020-10-01")
In order to make the data easier to work with, combine the tiles into a long dataframe with each row representing one tile in one quarter. The geometry now represents the NUTS region, not the original tile shape.
tiles_all <- tiles_q1_nuts %>%
rbind(tiles_q2_nuts) %>%
rbind(tiles_q3_nuts) %>%
rbind(tiles_q4_nuts) %>%
mutate(quarter_start = ymd(quarter_start)) # convert to date format
With this dataframe, you can start to generate some aggregates. In this table you’ll include the tile count, test count, quarter and average download and upload speeds.
Exploratory data analysis
aggs_quarter <- tiles_all %>%
st_set_geometry(NULL) %>%
group_by(quarter_start) %>%
summarise(tiles = n(),
avg_d_mbps = weighted.mean(avg_d_kbps / 1000, tests), # I find Mbps easier to work with
avg_u_mbps = weighted.mean(avg_u_kbps / 1000, tests),
tests = sum(tests)) %>%
ungroup()
knitr::kable(aggs_quarter) %>%
kable_styling()
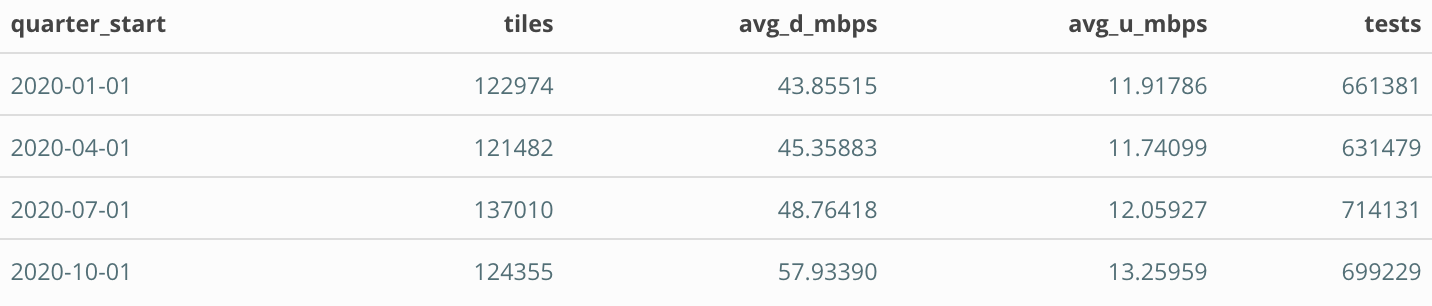
We can see from this table that both download and upload speeds increased throughout the year, with a small dip in upload speeds in Q2. Next, you’ll want to plot this data.
ggplot(aggs_quarter, aes(x = quarter_start)) +
geom_point(aes(y = avg_d_mbps), color = purple) +
geom_line(aes(y = avg_d_mbps), color = purple, lwd = 0.5) +
geom_text(aes(y = avg_d_mbps - 2, label = round(avg_d_mbps, 1)), color = purple, size = 3, family = "sans") +
geom_text(data = NULL, x = ymd("2020-02-01"), y = 47, label = "Download speed", color = purple, size = 3, family = "sans") +
geom_point(aes(y = avg_u_mbps), color = light_purple) +
geom_line(aes(y = avg_u_mbps), color = light_purple, lwd = 0.5) +
geom_text(aes(y = avg_u_mbps - 2, label = round(avg_u_mbps, 1)), color = light_purple, size = 3, family = "sans") +
geom_text(data = NULL, x = ymd("2020-02-05"), y = 14, label = "Upload speed", color = light_purple, size = 3, family = "sans") +
labs(y = "", x = "Quarter start date",
title = "Mobile Network Performance, U.K.",
subtitle = "Ookla® Open Datasets | 2020") +
theme(panel.grid.minor = element_blank(),
panel.grid.major = element_blank(),
axis.title.x = element_text(hjust=1)) +
scale_y_continuous(labels = label_number(suffix = " Mbps", scale = 1, accuracy = 1)) +
scale_x_date(date_labels = "%b %d")
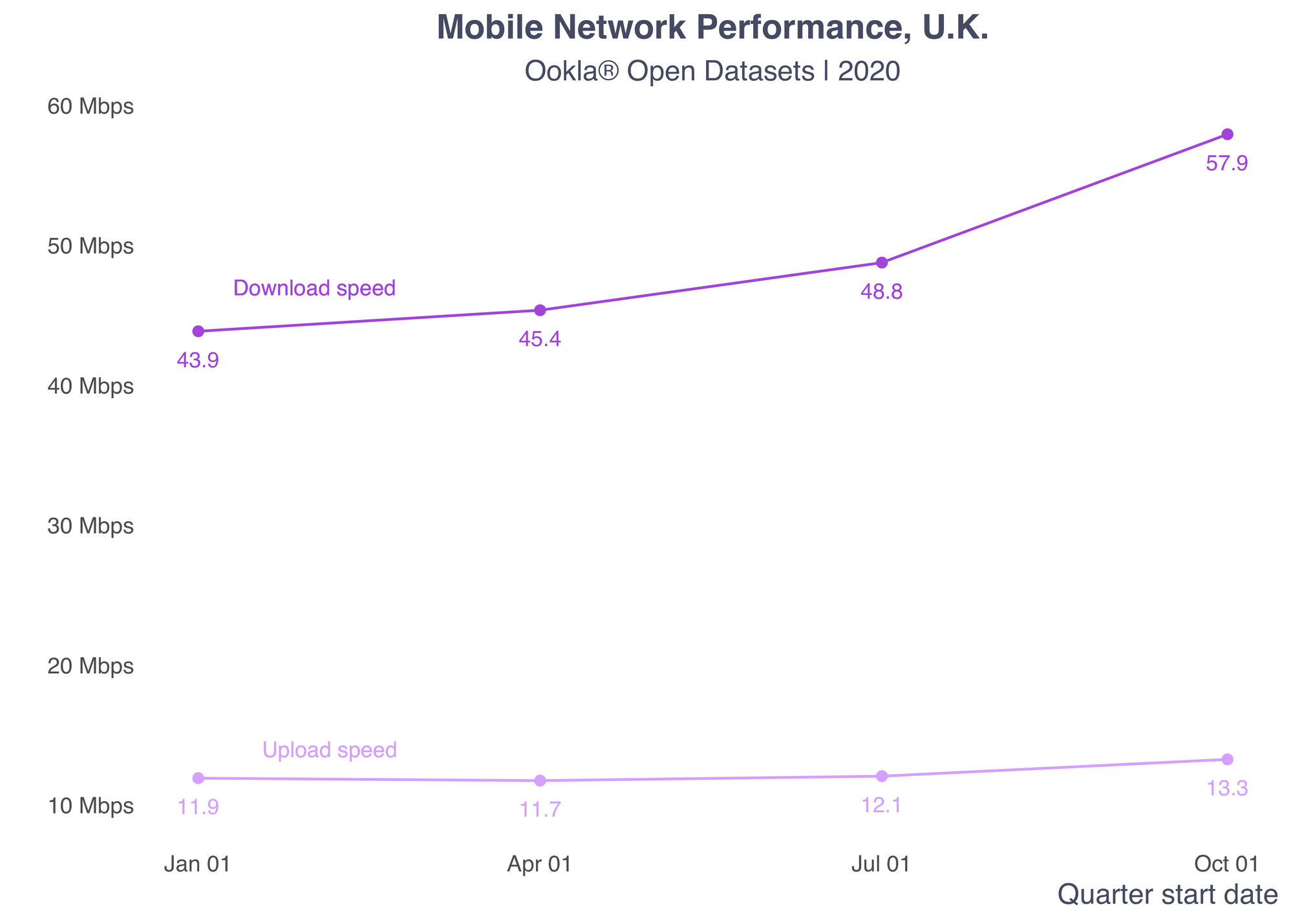
Examining test counts
We also saw above that the number of tests decreased between Q1 and Q2 and then peaked in Q3 at a little over 700,000 before coming back down. The increase likely followed resulted from interest in network performance during COVID-19 when more people started working from home. This spike is even more obvious in chart form.
ggplot(aggs_quarter, aes(x = quarter_start)) +
geom_point(aes(y = tests), color = purple) +
geom_line(aes(y = tests), color = purple, lwd = 0.5) +
geom_text(aes(y = tests - 6000, label = comma(tests), x= quarter_start + 5), size = 3, color = purple) +
labs(y = "", x = "Quarter start date",
title = "Mobile Test Count, U.K.",
subtitle = "Ookla® Open Datasets | 2020") +
theme(panel.grid.minor = element_blank(),
panel.grid.major = element_blank(),
axis.title.x = element_text(hjust=1),
axis.text = element_text(color = blue_gray)) +
scale_y_continuous(labels = comma) +
scale_x_date(date_labels = "%b %d")
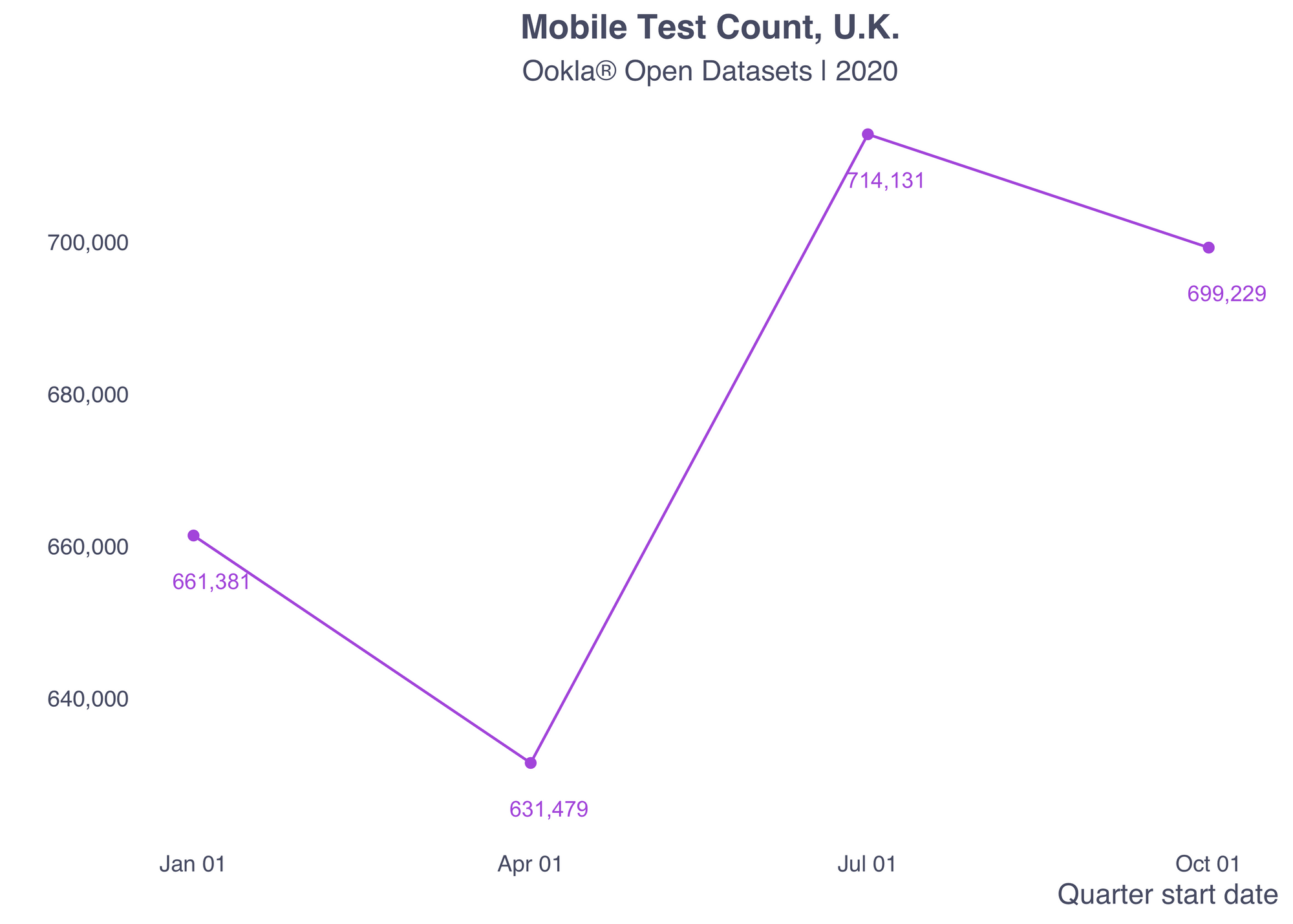
Data distribution
Next, I wanted to check the distribution of average download speeds.
ggplot(tiles_all) +
geom_histogram(aes(x = avg_d_kbps / 1000, group = quarter_start), size = 0.3, color = light_gray, fill = green) +
scale_x_continuous(labels = label_number(suffix = " Mbps", accuracy = 1)) +
scale_y_continuous(labels = comma) +
facet_grid(quarter_start ~ .) +
theme(panel.grid.minor = element_blank(),
panel.grid.major = element_blank(),
axis.title.x = element_text(hjust=1),
axis.text = element_text(color = blue_gray),
strip.text.y = element_text(angle = 0, color = blue_gray)) +
labs(y = "", x = "", title = "Mobile Download Speed Distribution by Tile, U.K.",
subtitle = "Ookla® Open Datasets | 2020")
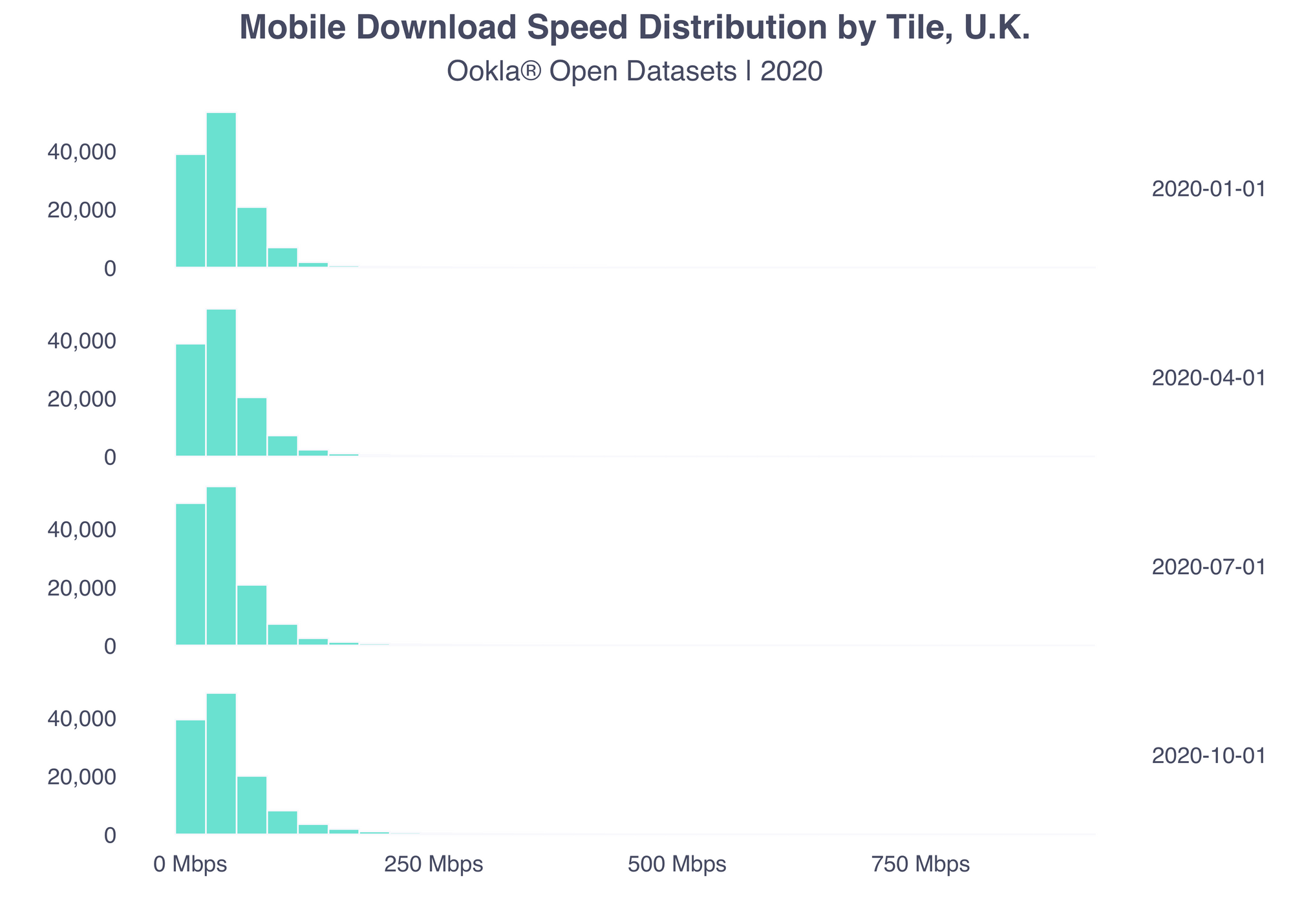
The underlying distribution of average download speeds across the tiles has stayed fairly stable.
Mapping average speed
Making a quick map of the average download speed in each region across the U.K. is relatively simple.
# generate aggregates table
nuts_3_aggs <- tiles_all %>%
group_by(quarter_start, nuts_id, nuts_name, urbn_desc, urbn_type) %>%
summarise(tiles = n(),
avg_d_mbps = weighted.mean(avg_d_kbps / 1000, tests), # I find Mbps easier to work with
avg_u_mbps = weighted.mean(avg_u_kbps / 1000, tests),
tests = sum(tests)) %>%
ungroup()
ggplot(nuts_3_aggs %>% filter(quarter_start == "2020-10-01")) +
geom_sf(aes(fill = avg_d_mbps), color = blue_gray, lwd = 0.08) +
scale_fill_stepsn(colors = RColorBrewer::brewer.pal(n = 5, name = "BuPu"), labels = label_number(suffix = " Mbps"), n.breaks = 4, guide = guide_colorsteps(title = "")) +
theme(panel.grid.minor = element_blank(),
panel.grid.major = element_blank(),
axis.title.x = element_text(hjust=1),
legend.text = element_text(color = blue_gray),
axis.text = element_blank()) +
labs(title = "Mobile Download Speed, U.K.", subtitle = "Ookla® Open Datasets | Q4 2020")
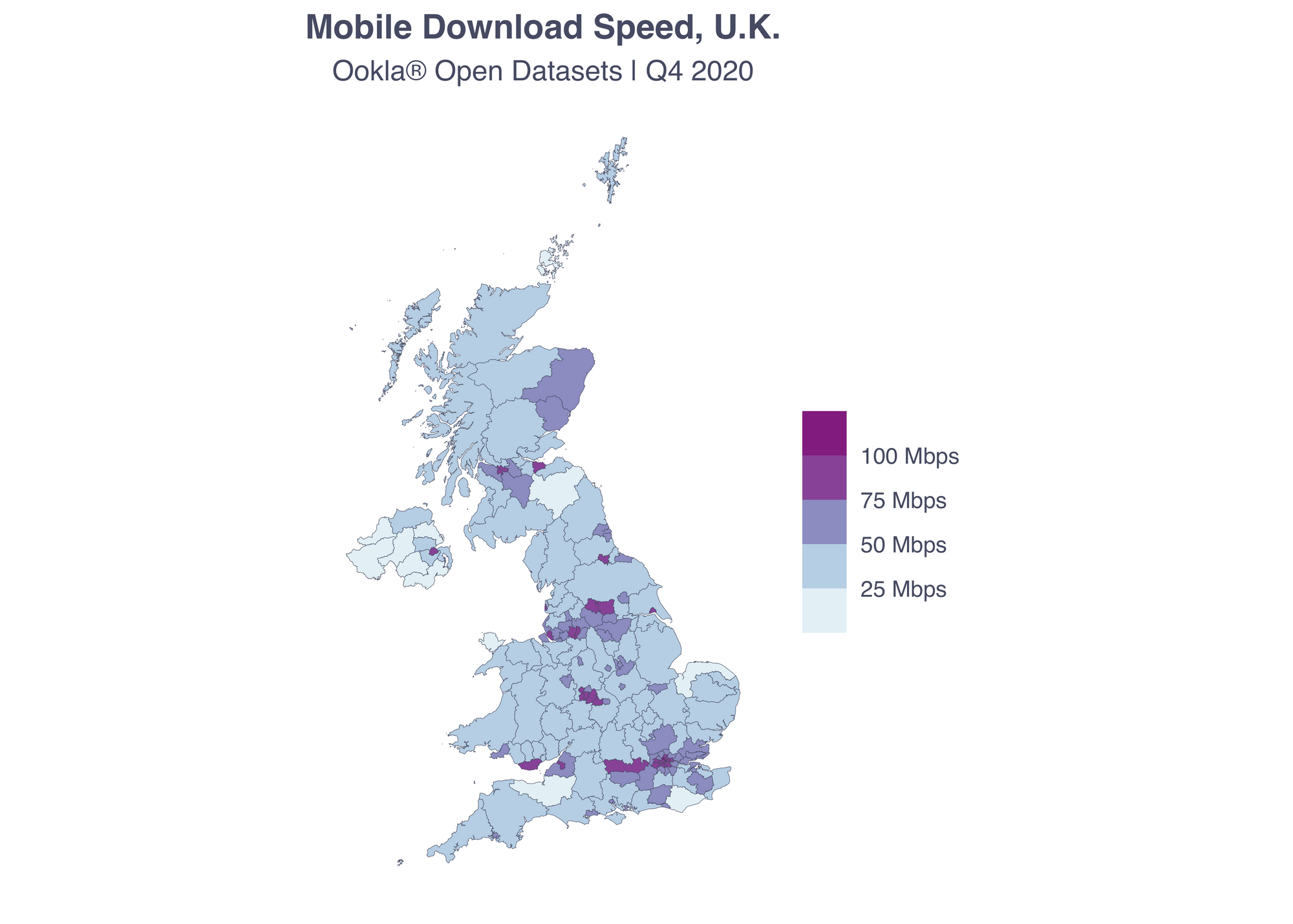
As you can see, the areas around large cities have faster download speeds on average and the lowest average download speeds are typically in more rural areas.
Rural and urban analysis
People are often interested in the difference between mobile networks in urban and rural areas. The Eurostat NUTS data includes an urban indicator with three levels: rural, intermediate and urban. This typology is determined primarily by population density and proximity to a population center.
ggplot(uk_nuts_3) +
geom_sf(aes(fill = urbn_desc), color = light_gray, lwd = 0.08) +
geom_text_repel(data = uk_cities,
aes(label = city_name, geometry = geometry),
family = "sans",
color = "#1a1b2e",
size = 2.2,
stat = "sf_coordinates",
min.segment.length = 2) +
scale_fill_manual(values = c(purple, light_purple, green), name = "", guide = guide_legend(direction = "horizontal", label.position = "top", keywidth = 3, keyheight = 0.5)) +
labs(title = "U.K., NUTS 3 Areas") +
theme(panel.grid.major = element_blank(),
panel.grid.minor = element_blank(),
axis.text = element_blank(),
axis.title = element_blank(),
legend.position = "top")
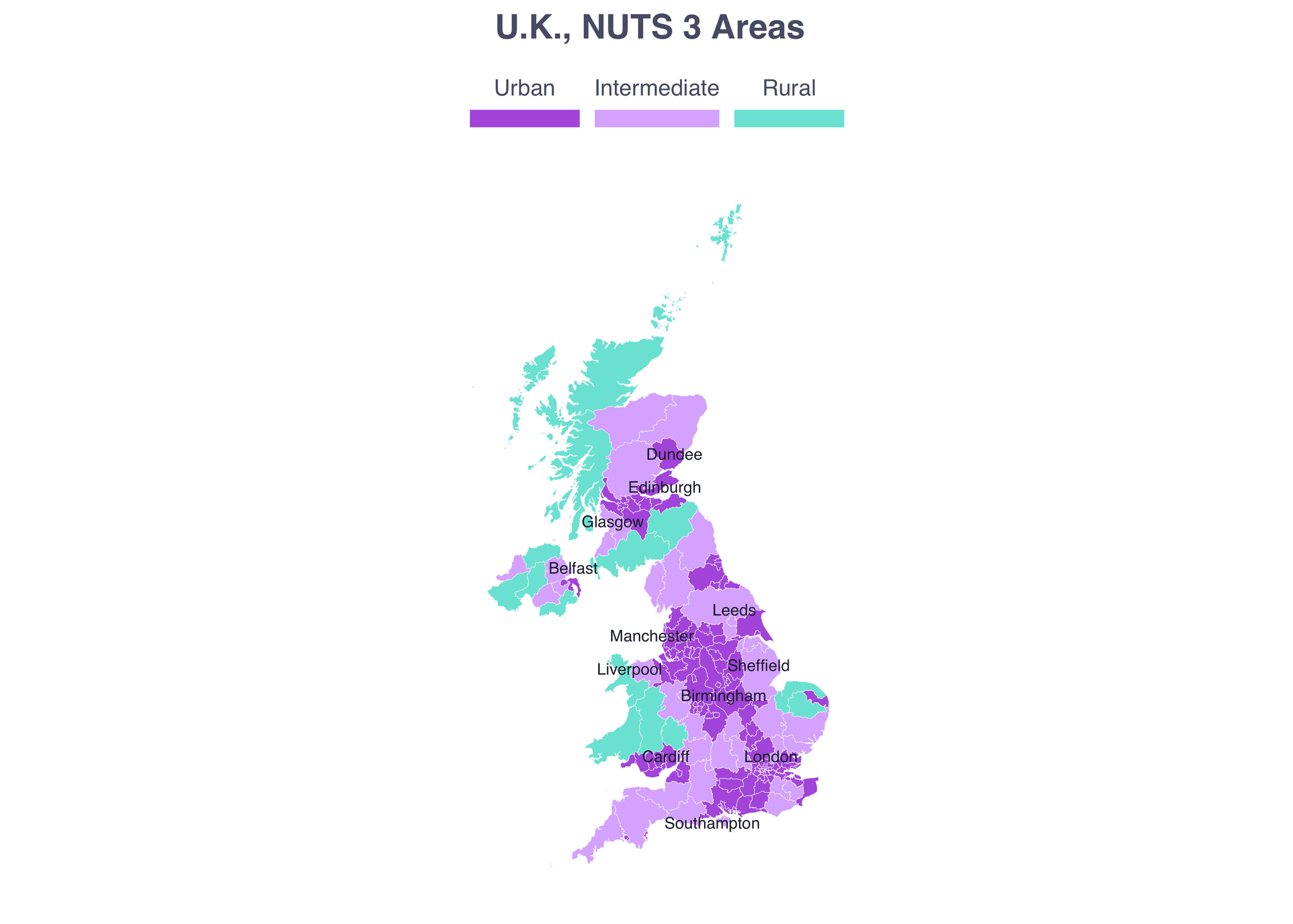
Data distribution overall and over time
When you aggregate by the urban indicator variable different patterns come up in the data.
# generate aggregates table
rural_urban_aggs <- tiles_all %>%
st_set_geometry(NULL) %>%
group_by(quarter_start, urbn_desc, urbn_type) %>%
summarise(tiles = n(),
avg_d_mbps = weighted.mean(avg_d_kbps / 1000, tests), # I find Mbps easier to work with
avg_u_mbps = weighted.mean(avg_u_kbps / 1000, tests),
tests = sum(tests)) %>%
ungroup()
As you might expect, the download speeds during Q4 are faster in urban areas than in rural areas – with the intermediate ones somewhere in between. This pattern holds for other quarters as well.
ggplot(rural_urban_aggs %>% filter(quarter_start == "2020-10-01"), aes(x = avg_d_mbps, y = urbn_desc, fill = urbn_desc)) +
geom_col(width = .3, show.legend = FALSE) +
geom_jitter(data = nuts_3_aggs, aes(x = avg_d_mbps, y = urbn_desc, color = urbn_desc), size = 0.7) +
geom_text(aes(x = avg_d_mbps - 4, label = round(avg_d_mbps, 1)), family = "sans", size = 3.5, color = blue_gray) +
scale_fill_manual(values = c(purple, light_purple, green)) +
scale_color_manual(values = darken(c(purple, light_purple, green))) +
scale_x_continuous(labels = label_number(suffix = " Mbps", scale = 1, accuracy = 1)) +
theme(panel.grid.minor = element_blank(),
panel.grid.major = element_blank(),
axis.title.x = element_text(hjust=1),
legend.position = "none",
axis.text = element_text(color = blue_gray)) +
labs(y = "", x = "",
title = "Mobile Download Speed Distribution by NUTS 3 Area, U.K.",
subtitle = "Ookla® Open Datasets | 2020")
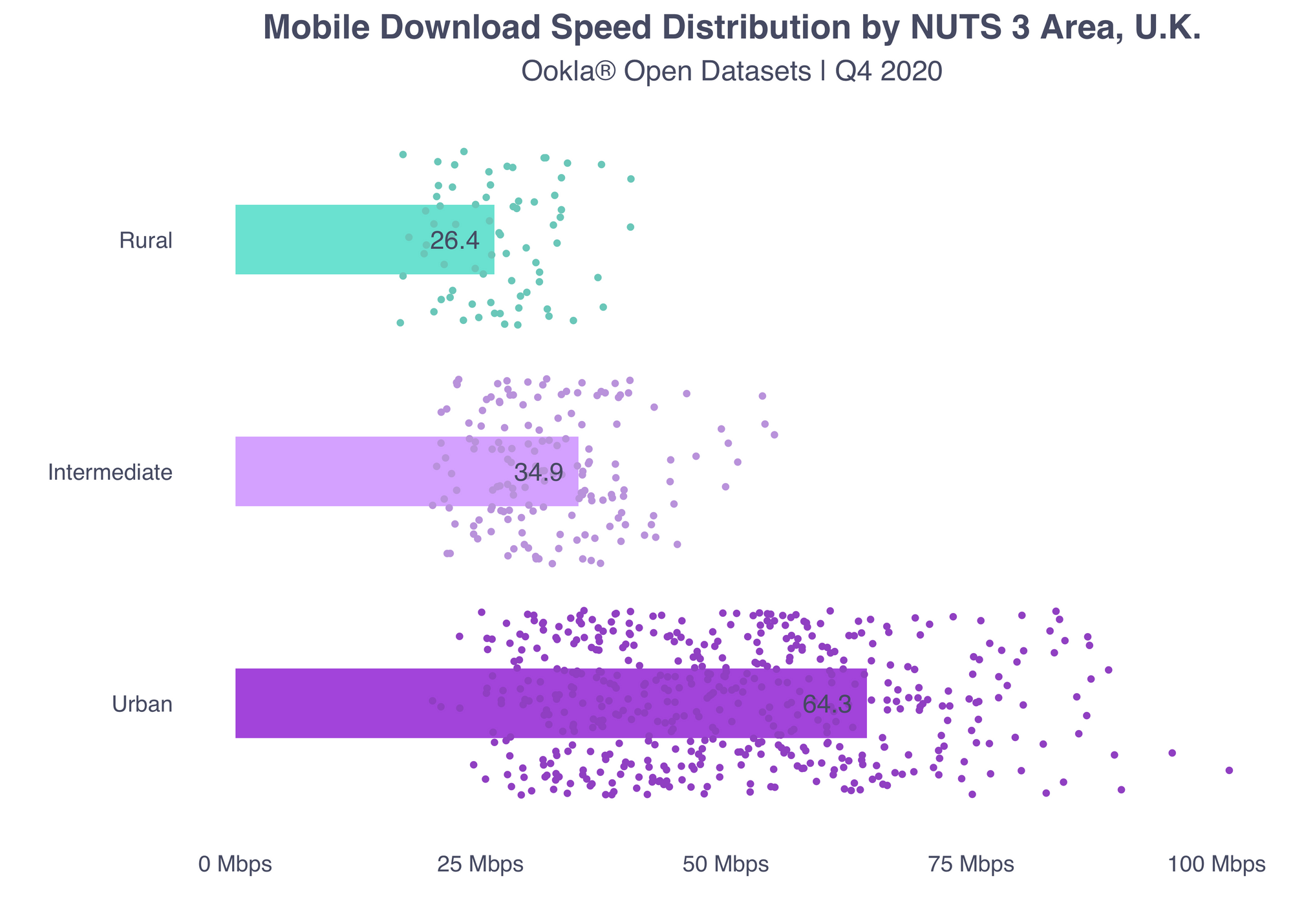
Interestingly though, the patterns differ when you look at a time series plot. Urban mobile networks steadily improve, while the intermediate and rural areas saw slower average download speeds starting in Q2 before going back up after Q3. This is likely the result of increased pressure on the networks during stay-at-home orders (although this graph is not conclusive evidence of that).
ggplot(rural_urban_aggs) +
geom_line(aes(x = quarter_start, y = avg_d_mbps, color = urbn_desc)) +
geom_point(aes(x = quarter_start, y = avg_d_mbps, color = urbn_desc)) +
# urban label
geom_text(data = NULL, x = ymd("2020-02-01"), y = 50, label = "Urban", color = purple, family = "sans", size = 3) +
# intermediate label
geom_text(data = NULL, x = ymd("2020-02-15"), y = 35, label = "Intermediate", color = light_purple, family = "sans", size = 3) +
# rural label
geom_text(data = NULL, x = ymd("2020-01-15"), y = 26, label = "Rural", color = green, family = "sans", size = 3) +
scale_color_manual(values = c(purple, light_purple, green)) +
scale_x_date(date_labels = "%b %d") +
scale_y_continuous(labels = label_number(suffix = " Mbps", scale = 1, accuracy = 1)) +
theme(panel.grid.minor = element_blank(),
panel.grid.major = element_blank(),
axis.title.x = element_text(hjust=1),
legend.position = "none",
axis.text = element_text(color = blue_gray)) +
labs(y = "", x = "Quarter start date",
title = "Mobile Download Speed by NUTS 3 Urban-Rural Type, U.K.",
subtitle = "Ookla® Open Datasets | 2020")
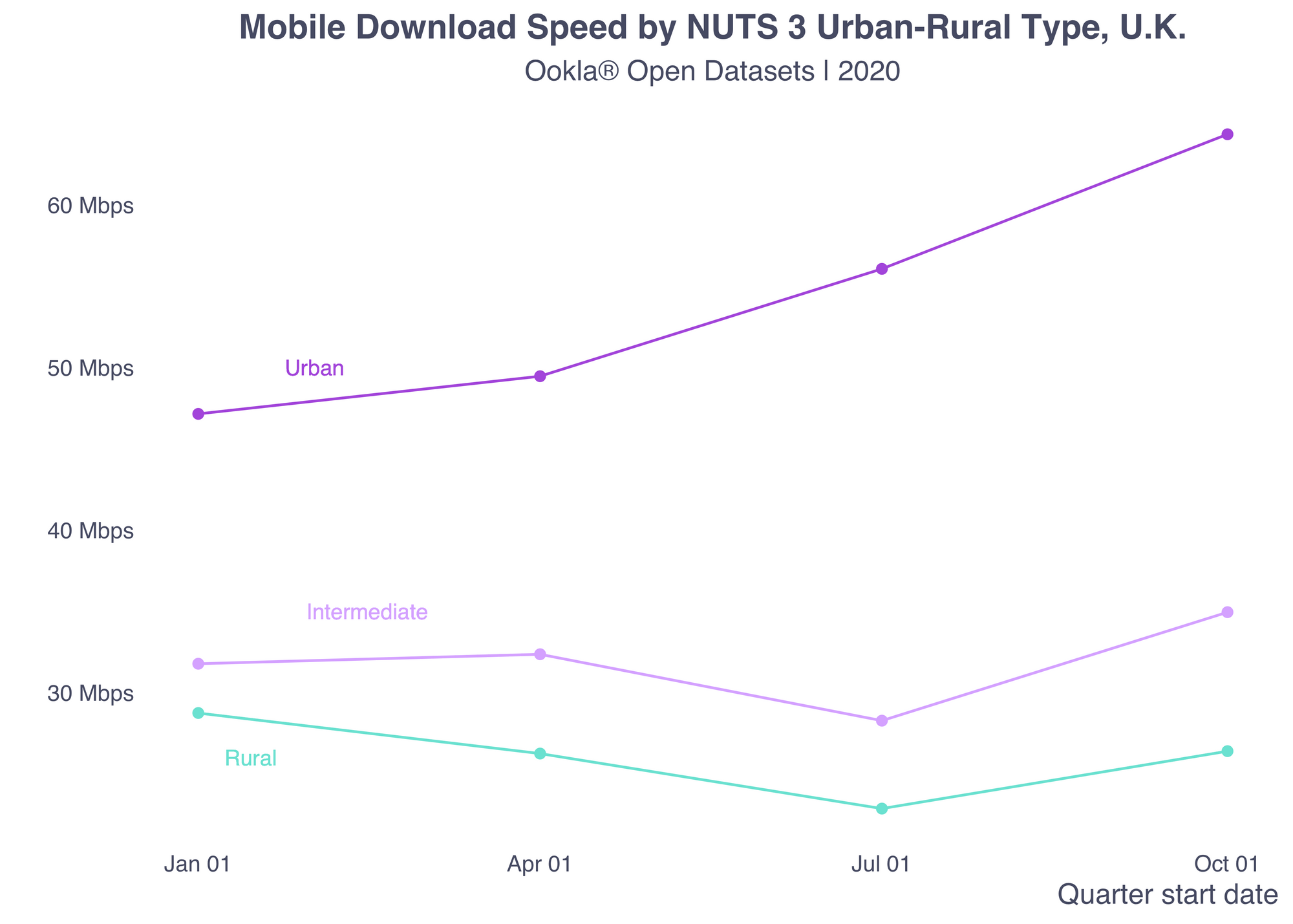
When you repeat the same plot but map the test count to the site of the point, you can see why the overall download speed increased steadily. The number of tests in urban areas is much higher than in intermediate and rural areas, thus pulling up the overall average.
ggplot(rural_urban_aggs) +
geom_line(aes(x = quarter_start, y = avg_d_mbps, color = urbn_desc)) +
geom_point(aes(x = quarter_start, y = avg_d_mbps, color = urbn_desc, size = tests)) +
# urban label
geom_text(data = NULL, x = ymd("2020-02-01"), y = 50, label = "Urban", color = purple, family = "sans", size = 3) +
# intermediate label
geom_text(data = NULL, x = ymd("2020-02-15"), y = 35, label = "Intermediate", color = light_purple, family = "sans", size = 3) +
# rural label
geom_text(data = NULL, x = ymd("2020-01-15"), y = 26, label = "Rural", color = green, family = "sans", size = 3) +
scale_color_manual(values = c(purple, light_purple, green)) +
scale_x_date(date_labels = "%b %d") +
scale_y_continuous(labels = label_number(suffix = " Mbps", scale = 1, accuracy = 1)) +
theme(panel.grid.minor = element_blank(),
panel.grid.major = element_blank(),
axis.title.x = element_text(hjust=1),
legend.position = "none",
axis.text = element_text(color = blue_gray)) +
labs(y = "", x = "Quarter start date",
title = ("Mobile Download Speed by NUTS 3 Urban-Rural Type, U.K."),
subtitle = "Ookla® Open Datasets | 2020",
caption = "Circle size indicates test count")
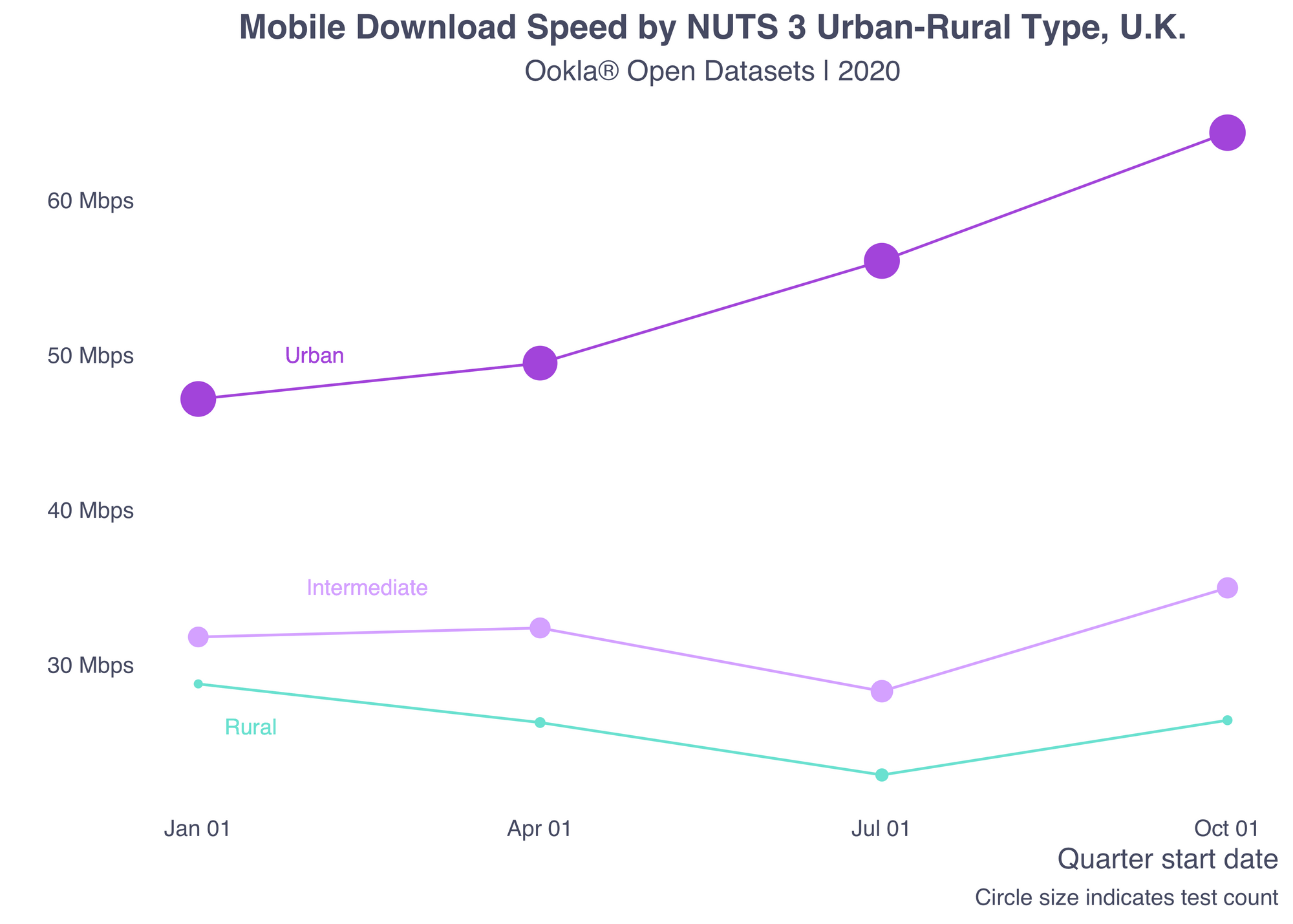
Spotlighting regional variances
Parsing the data by specific geographies can reveal additional information.
bottom_20_q4 <- nuts_3_aggs %>%
filter(quarter_start == "2020-10-01") %>%
top_n(n = -20, wt = avg_d_mbps) %>%
mutate(nuts_name = fct_reorder(factor(nuts_name), -avg_d_mbps))
map <- ggplot() +
geom_sf(data = uk_nuts_3, fill = light_gray, color = mid_gray, lwd = 0.08) +
geom_sf(data = bottom_20_q4, aes(fill = urbn_desc), color = mid_gray, lwd = 0.08, show.legend = FALSE) +
geom_text_repel(data = uk_cities,
aes(label = city_name, geometry = geometry),
family = "sans",
color = blue_gray,
size = 2.2,
stat = "sf_coordinates",
min.segment.length = 2) +
scale_fill_manual(values = c(purple, light_purple, green), name = "", guide = guide_legend(direction = "horizontal", label.position = "top", keywidth = 3, keyheight = 0.5)) +
labs(title = NULL,
subtitle = NULL) +
theme(panel.grid.major = element_blank(),
panel.grid.minor = element_blank(),
axis.text = element_blank(),
axis.title = element_blank(),
legend.position = "top")
barplot <- ggplot(data = bottom_20_q4, aes(x = avg_d_mbps, y = nuts_name, fill = urbn_desc)) +
geom_col(width = .5) +
scale_fill_manual(values = c(purple, light_purple, green), guide = guide_legend(direction = "horizontal", label.position = "top", keywidth = 3, keyheight = 0.5, title = NULL)) +
scale_x_continuous(labels = label_number(suffix = " Mbps", scale = 1, accuracy = 1)) +
theme(panel.grid.minor = element_blank(),
panel.grid.major = element_blank(),
axis.title.x = element_text(hjust=1),
legend.position = "top",
axis.text = element_text(color = blue_gray)) +
labs(y = "", x = "",
title = ("Slowest 20 NUTS 3 Areas by Download Speed, U.K."),
subtitle = "Ookla® Open Datasets | Q4 2020")
# use patchwork to put it all together
barplot + map
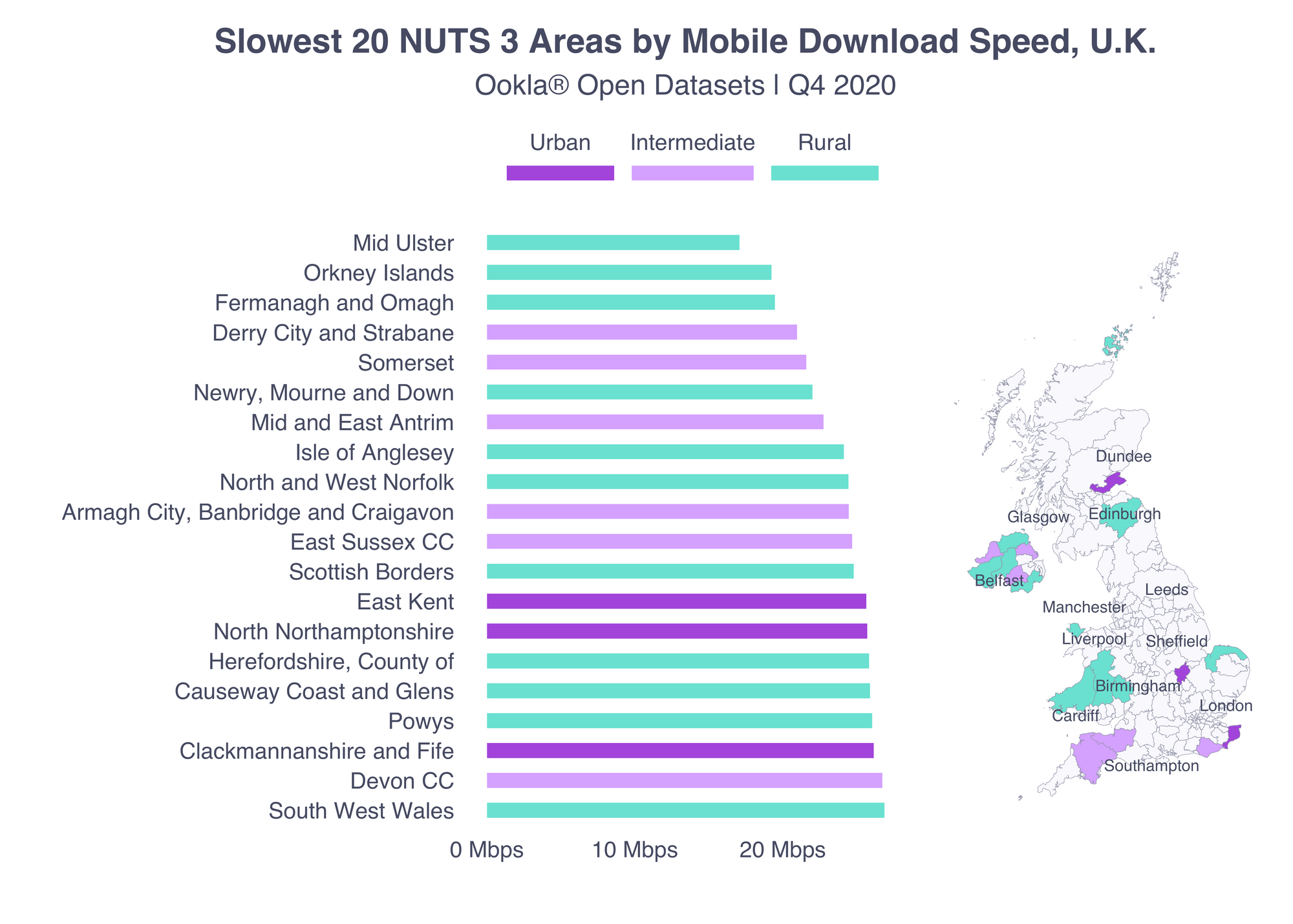
Among the 20 areas with the lowest average download speed in Q4 2020 there were three urban areas and six intermediate. The rest were rural.
top_20_q4 <- nuts_3_aggs %>%
filter(quarter_start == "2020-10-01") %>%
top_n(n = 20, wt = avg_d_mbps) %>%
mutate(nuts_name = fct_reorder(factor(nuts_name), avg_d_mbps))
top_map <- ggplot() +
geom_sf(data = uk_nuts_3, fill = light_gray, color = mid_gray, lwd = 0.08) +
geom_sf(data = top_20_q4, aes(fill = urbn_desc), color = mid_gray, lwd = 0.08, show.legend = FALSE) +
geom_text_repel(data = uk_cities,
aes(label = city_name, geometry = geometry),
family = "sans",
color = blue_gray,
size = 2.2,
stat = "sf_coordinates",
min.segment.length = 2) +
scale_fill_manual(values = c(purple, light_purple, green), name = "", guide = guide_legend(direction = "horizontal", label.position = "top", keywidth = 3, keyheight = 0.5)) +
labs(title = NULL,
subtitle = NULL) +
theme(panel.grid.major = element_blank(),
panel.grid.minor = element_blank(),
axis.text = element_blank(),
axis.title = element_blank(),
legend.position = "top")
top_barplot <- ggplot(data = top_20_q4, aes(x = avg_d_mbps, y = nuts_name, fill = urbn_desc)) +
geom_col(width = .5) +
scale_fill_manual(values = c(purple, light_purple, green), guide = guide_legend(direction = "horizontal", label.position = "top", keywidth = 3, keyheight = 0.5, title = NULL)) +
scale_x_continuous(labels = label_number(suffix = " Mbps", scale = 1, accuracy = 1), breaks = c(50, 100)) +
theme(panel.grid.minor = element_blank(),
panel.grid.major = element_blank(),
axis.title.x = element_text(hjust=1),
legend.position = "top",
axis.text = element_text(color = blue_gray)) +
labs(y = "", x = "",
title = "Fastest 20 NUTS 3 Areas by Mobile Download Speed, U.K.",
subtitle = "Ookla® Open Datasets | Q4 2020")
top_london <- ggplot() +
geom_sf(data = uk_nuts_3 %>% filter(str_detect(fid, "UKI")), fill = light_gray, color = mid_gray, lwd = 0.08) +
geom_sf(data = top_20_q4 %>% filter(str_detect(nuts_id, "UKI")), aes(fill = urbn_desc), color = mid_gray, lwd = 0.08, show.legend = FALSE) +
geom_text_repel(data = uk_cities %>% filter(city_name == "London"),
aes(label = city_name, geometry = geometry),
family = "sans",
color = "black",
size = 2.2,
stat = "sf_coordinates",
min.segment.length = 2) +
scale_fill_manual(values = c(purple, light_purple, green), name = "", guide = guide_legend(direction = "horizontal", label.position = "top", keywidth = 3, keyheight = 0.5)) +
labs(title = NULL,
subtitle = NULL) +
theme(panel.grid.major = element_blank(),
panel.grid.minor = element_blank(),
axis.text = element_blank(),
axis.title = element_blank(),
legend.position = "top",
panel.border = element_rect(colour = blue_gray, fill=NA, size=0.5))
top_map_comp <- top_map + inset_element(top_london, left = 0.6, bottom = 0.6, right = 1, top = 1)
top_barplot + top_map_comp
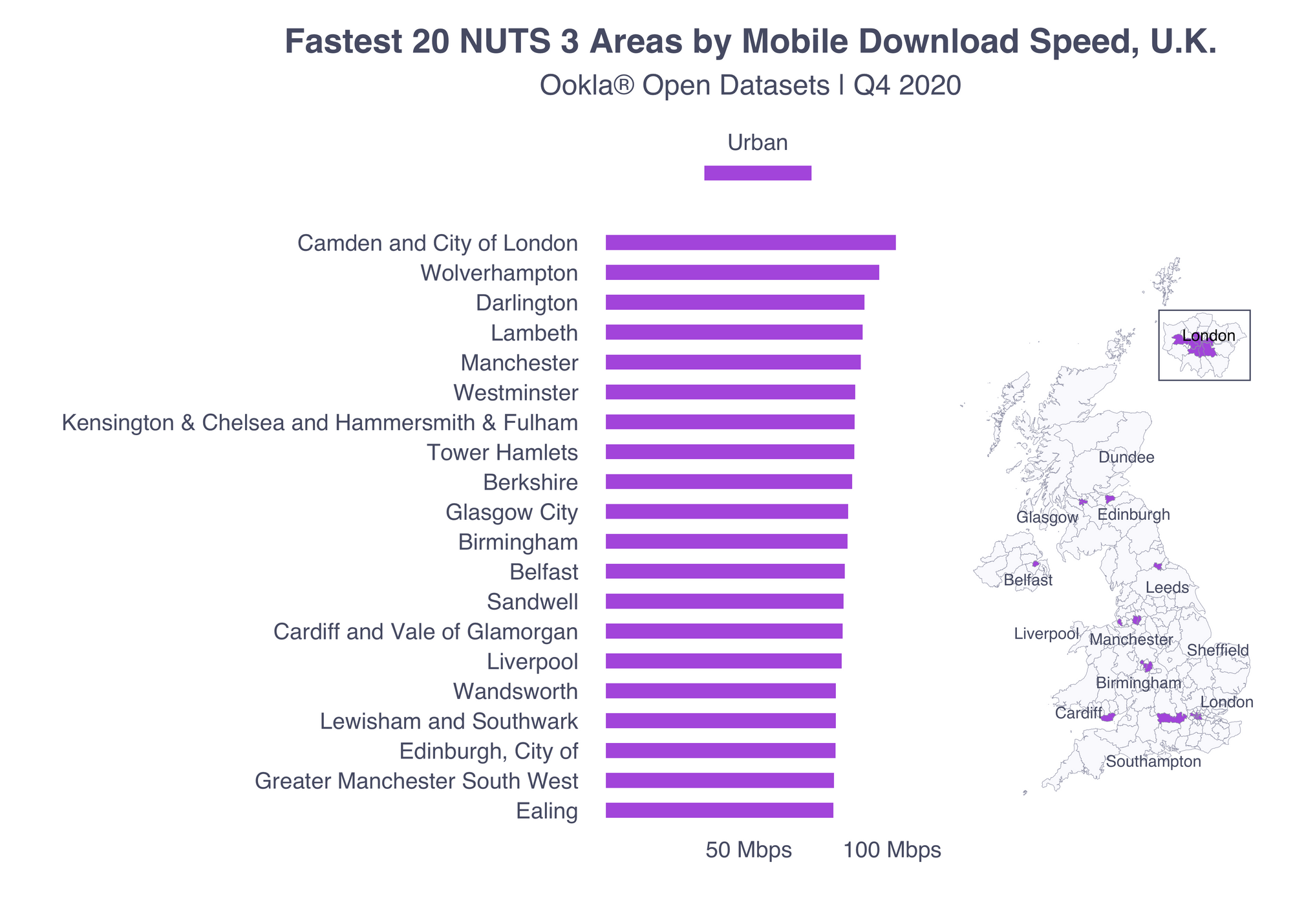
Meanwhile, all of the fastest 20 NUTS 3 areas were urban.
What else you can do with this data
Don’t forget there are also more tutorials with examples written in Python and R. Aside from what I showed here, you could do an interesting analysis looking at clustering patterns, sociodemographic variables and other types of administrative units like legislative or school districts.
We hope this tutorial will help you use Ookla’s open data for your own projects. Please tag us if you share your projects on social media using the hashtag #OoklaForGood so we can learn from your analyses.